What is Audience Data Bias? Here is Why You Should Care
Have you ever looked at audience data and thought that it does not seem completely real or accurate? It could be a result of data bias. Data bias is when the source data is skewed, providing results that are not fully representative of the audience you are researching, and can be either intentionally or unintentionally done. Either way, data bias is something to be taken into account in your planning and strategy.
Before jumping in further, you might want to read our 2-post series on how we use and enrich our data source at Audiense, and the data restrictions that apply to everyone, and how this works in the real world.
An example of data bias can be found quite simply in demographic and socio-economic data. Gender population in India is 52% men, 48% women. When it comes to social data, for starters, the internet penetration of the population is at 49%, and then when we look at the India population within Facebook Insights, it tells us that the gender divide is 76% men, 24% women! So, what is accurate? This shows us that there is an unbalance between how many men and women are on social media, despite how many men and women there are in the country. Simply put, we know that the entire adult population of the world isn’t on social media, so we know that the data we are working with is only going to be representative of the social media population. If we want to go really deep, we need to remember that people can create multiple social accounts, such as private accounts, or fan pages, and this can differ depending on the online community you are analysing.
Making sure that private data is not accessible
The difference between Facebook and Twitter data sources, and what is publicly available, is that Facebook had left the floodgates open from the start, so everything was exposed and for the taking, and once it was out there, they could not reclaim it. Whereas, Twitter, in building their platform and database were already putting in processes of safeguarding personal data, which means the access they allow businesses, like us, to use is compliant from the start. Twitter’s availability of an API allows Audiense to view all the available, compliant, public data via a direct stream. Via Twitter’s use of their APIs, such as Gnip, they make sure that when data is deleted, or an account turns private, that the data cannot be accessed again by data partners, or they notify the partners, like us, so we can delete it within our access.
Without a complete API, it leaves the door open for other methods, whether this is reduced API access, or even data scraping. There are other networks with a lower level of API, more so for you to understand your own analytics. This includes Instagram through InfluencerDB. InfluencerDB was a popular influencer community management tool, which used a combination of scraping and users opting-in to be able to view their stats, but has recently announced its closing.
There are some networks with no APIs, which are still data providers. For example, TikTok, which is then scraped and ‘licensed’ by platforms such as Influencer Grid, Netfeedr, and Pentos to provide information on TikTok influencers and analytics. The difficulty TikTok might have is that it’s platform has a large amount of children using, creating, and sharing within the app, and so they have a responsibility to protect its users and their data from harmful practices.
All of the above networks and platforms might apply additional machine learning to further understand and analyze the data collected. Similarly, some providers might do either heavy sampling and extrapolation, or cross-network matching and extrapolation.
Audiense does not scrape data
Then there is the issue of data scraping itself causing data bias. Audiense does not scrape data. We have access to the Twitter API, so we know all our data is as accurate as people portray themselves to be on that platform. However, other platforms that are weighting their data source through restricted platforms, such as Facebook and Instagram, will be relying on scraped data. (Facebook sued a couple of companies recently for the level in which they carried this out!) This means that they won’t have 100% of the data, and will essentially be working with small and diluted sample sizes, which they then provide you, whilst you have the assumption this will create an accurate and successful campaign. Given it’s all that is available, it might be the best shot you have, but with the data being biased, your results still might fall short of your expectations when it comes to analysing an audience.
LinkedIn data is often seeked by audience intelligence platforms and their users. As a major platform for B2B, it is clear why people are keen to get whatever information they can, and why businesses want to be able to say they provide it. A well-liked tool which tries to bridge the gap is pipl.com, often used to help match handles to LinkedIn profiles. One case which went to court, was the dispute between LinkedIn and a small data analytics company, hiQ. Data scraping, depending on the circumstances, can either be legal or illegal. In that case, the data hiQ were scraping was public data, so using automated bots they were collecting data that anyone could view already. Phantombuster is another scraping tool, often used for scraping LinkedIn profile data.
Issues come into play when the data source itself, such as in the case of Cambridge Analytica and Facebook, is providing more public data than users might realistically be aware of happening. When considering a platform to use for your analytics, they may or may not be pushing the limits of what is ethical or allowed, and it will be your responsibility to use their data knowing that information. There are implications to consider, such as the accuracy and reliability of scraped data seeing as it will be based on sample sizes, but when you’re limited by the availability perhaps this is a compromise you must admit to acknowledging. How long will social data access, as we know it, last?
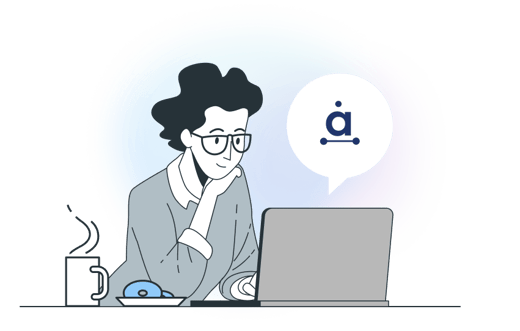
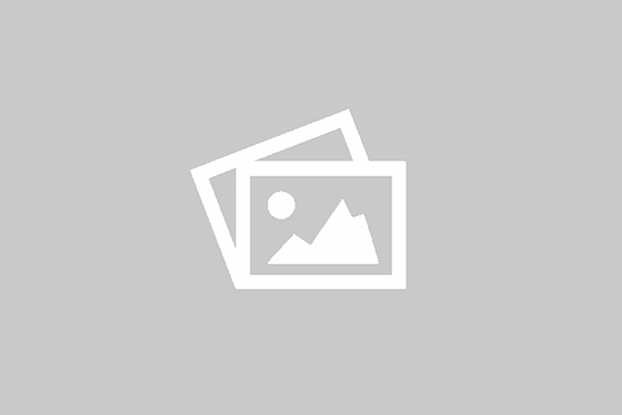